The Inside View
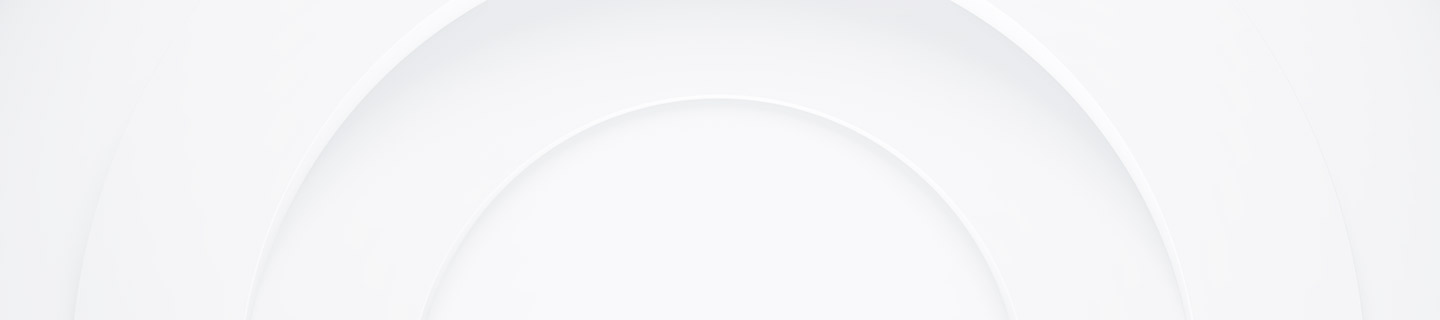
Hiroshi Nishiyuki highlights how IloT applications can deliver ‘visible’ results
First Published in Japanese in May 2021 [3 parts]
Part 1: Practical Use of IoT to Deliver Machine-shop Benefit
In factories, cutting tools as used in machine tools, are almost a consumable part. However, their performance and operational life can have a big impact on production costs, so keeping a close eye on their condition is no bad thing. In parallel, after all the hype around IoT and how it will revolutionize manufacturing, there are still relatively few practical use-cases with justifiable return-on-investment. Hiroshi Nishiyuki, Head of Mitsubishi Electric’s FA System Solutions Department, explains how the above two conditions converged and resulted in solving both problems.
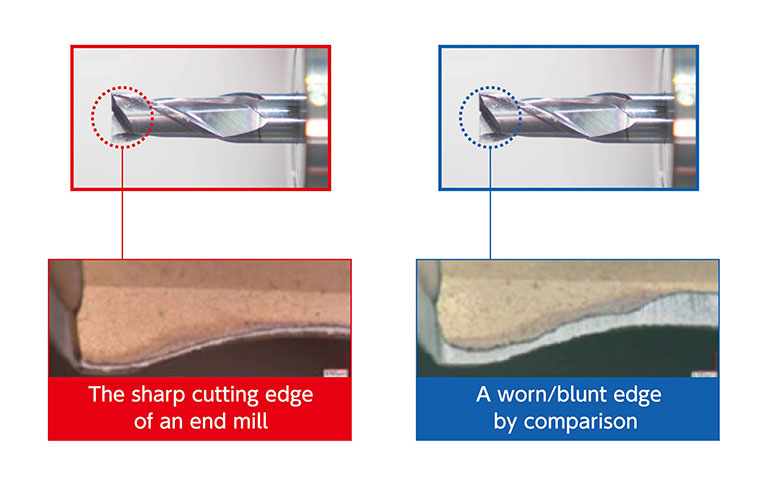
Electric shavers and machine tools have one thing in common - their cutters need replacing once in a while. Hiroshi Nishiyuki explains, “Sometimes I get lazy, especially on weekends and holidays, and I don't shave my beard. On Monday morning, I have a good shave to start the week off, but if I use the same blade for a long time, naturally it gets worn out.”
“While I was shaving one day, I remembered reading an old book reflecting on the fact that the beards of many men in the world grow every day, so the shaver industry is a very attractive business to be in,” he added. This view was based on the fact that the blades for electric shavers are consumable products, just like the toner used in copy machines.
The cutting tools used by machine tools are usually very expensive, unlike blades for electric shavers, so having lots of spare cutters is not economical. The trouble is that the condition of this consumable tooling has a lot to do with the quality of the machined parts. We can’t just let it pass like our bit of leftover beard saying, “Oh, it's a bad-beard day.” The best solution to keep your tooling sharp is to replace it frequently, but the cost cannot be ignored, and what’s more, you have to stop the machine every time you replace the tooling. So the question would be whether IoT can be used to solve this problem.
IoT is not the solution to everything
“It is not widely known that implementing IoT on factory floors is quite difficult,” says Nishiyuki. “What’s making things difficult to understand is the complexity of determining the return on investment.” Some of you may have tried using IoT in a proof of concept (PoC), but probably figured it would be too expensive and with little benefit.
There are several possible reasons for such failures, but one could be that there were no specific KPIs set before implementing the IoT solution. In this case, you might have initially believed that if you use the numerous amounts of data available and analyze that data, you will surely find something valuable. The fundamental problem is that the IoT solution is being introduced without any way to manage it through agreed KPIs.
Another case could be that IoT didn't bring the outcome initially expected even though a specific KPI was set. Such a KPI could have been, for example, the improvement in the yield of a product by a few percent resulting in reduced costs by a certain amount. IoT and AI are not the solution to everything. Where an NG (not good) product is made in manufacturing, there is a law of cause and effect. IoT and AI is about finding the relation between outcomes and factors that cause them. However, the discovery of such rules is often done by trial and error, which is a very labor-intensive and costly process. This means that, since no clear method has been established to improve yield, IoT investment for cost reduction may become an investment in research and development, causing cost overruns.
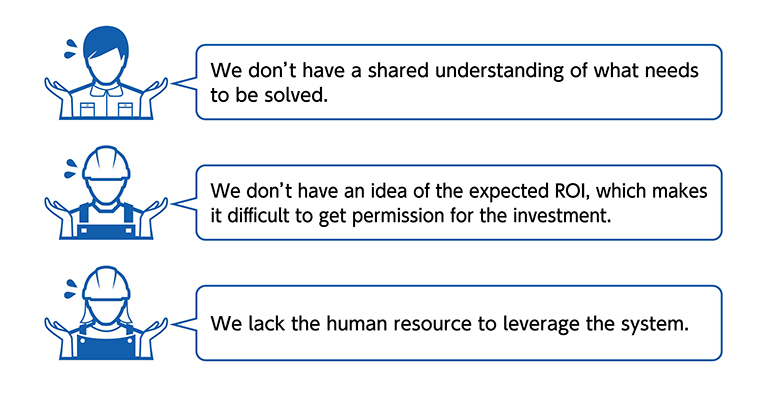
Is there a way to replace tooling without wasting money?
Going back to machine tools, if you want to use IoT to solve this problem, it is natural that you would want to introduce a proven solution.
The challenge starts there. Your first question would be, “Can we reduce machining defects and the frequency of tooling replacements at our factories, and how much investment do we need to do that?” As previously mentioned, you would need to figure out how much ROI you can expect before you decide on your IoT investment.
Nishiyuki explained, “We have years of experience in collecting and analyzing IoT data based on our manufacturing expertise and unique technologies. As a result, we have developed products that enable anyone to easily perform tooling management and quality control based on a quantitative standard.”
The solution is called the ‘iQ Monozukuri Machine Tool Wear Diagnosis,’ which is a diagnostic package for optimizing tooling management, addressing the questions of “When should we replace the tools?,” “Can we prevent sudden tool abnormalities?” or “How can we leverage data easily?”
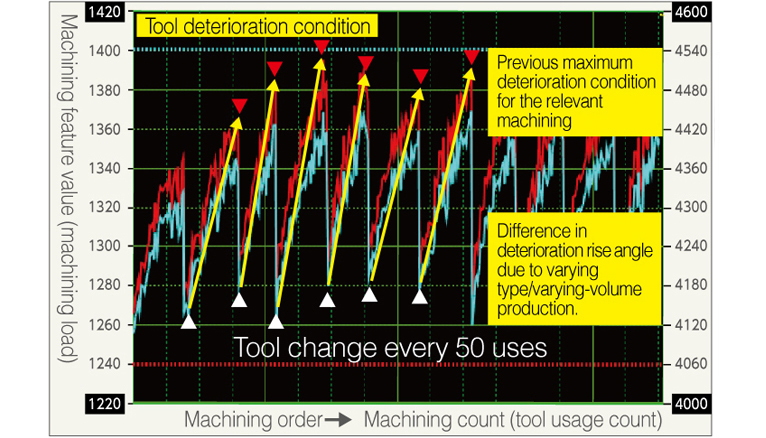

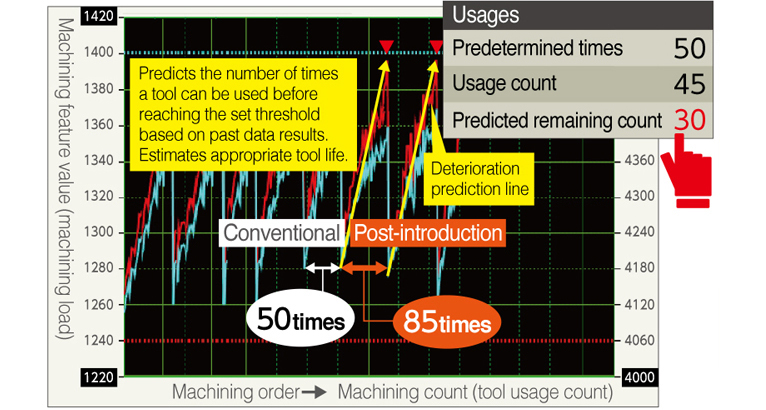
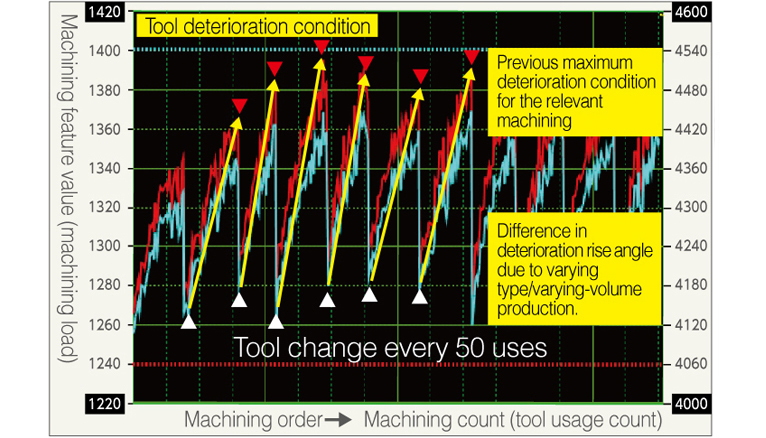

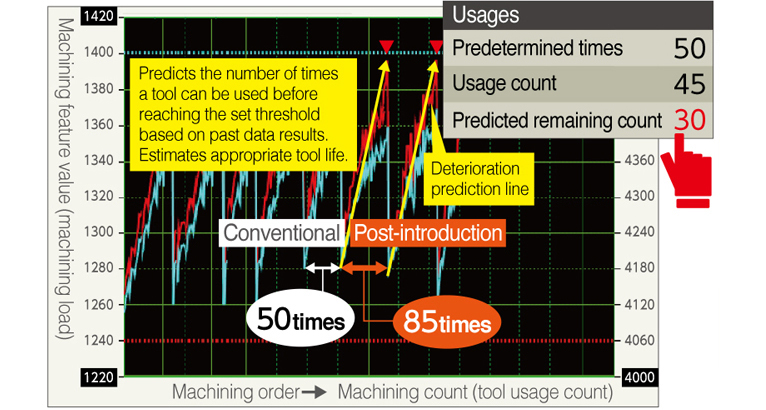
iQ Monozukuri Machine Tool Wear Diagnosis is a diagnostic package that can predict the number of times a tool can be used based on the condition of the tooling.
(Left) In the case of regularly scheduled replacements with time-based management (TBM), the tool was replaced based on the number of times it was used regardless of its condition, which often resulted in the tool not being used to its maximum lifespan.
(Right) With the iQ Monozukuri Machine Tool Wear Diagnosis replacements are decided with condition-based management (CBM), so the number of times the tool can be used is estimated based on the condition of the particular tool and the machining history data. The tool can be used for its full lifespan.
iQ Monozukuri Machine Tool Wear Diagnosis is a diagnostic package that can predict the number of times a tool can be used based on the condition of the tooling.
(Top) In the case of regularly scheduled replacements with time-based management (TBM), the tool was replaced based on the number of times it was used regardless of its condition, which often resulted in the tool not being used to its maximum lifespan.
(Bottom) With the iQ Monozukuri Machine Tool Wear Diagnosis replacements are decided with condition-based management (CBM), so the number of times the tool can be used is estimated based on the condition of the particular tool and the machining history data. The tool can be used for its full lifespan.
Customers who have already introduced this solution are now able to reduce the number of machining defects, and with some customers achieving a 40% reduction in annual tool cost, it has proved to bring significant ROI, which previously was a barrier for implementing an IoT solution.
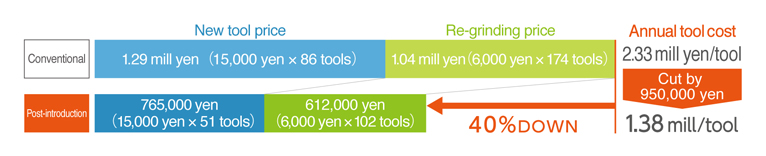
Nishiyuki concludes, “The current manufacturing environment with COVID-19 has made it difficult to produce products that rely on the experience and know-how of skilled workers, on the other hand the adoption of IoT is accelerating more than ever before. Therefore, for those who want to utilize IoT, or have already introduced it but have not yet found it effective, I highly recommend ‘iQ Monozukuri Machine Tool Wear Diagnosis’ as an IoT application package that will lead to tangible results.”
Products
- Summary
- Part 1: Practical Use of IoT to Deliver Machine-shop Benefit
- Part 2: Understanding the ROI of Visualization
- Part 3: Streamlining maintenance operations in factories reduces downtime and cost