Insights
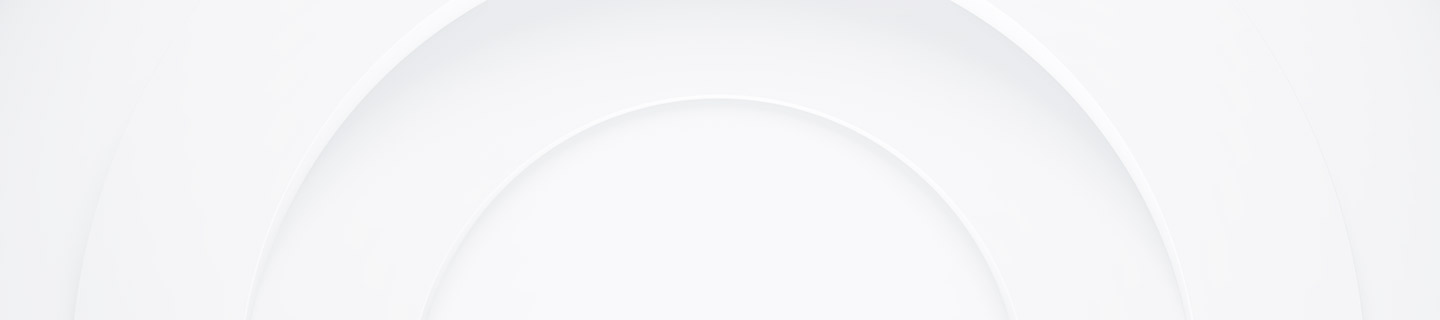
[MELSOFT MaiLab]Simple-to-use tool democratizes data analysis
First Published in Japanese in May 2023
As factories are being enabled for smart production, the quantity of data produced is increasing significantly. Unfortunately, there are times when there is so much data that it cannot be easily interpreted, and as a result manufacturing operations suffer. In order to resolve problems like this, Mitsubishi Electric has developed MELSOFT MaiLab, a data science tool which enables anyone to easily analyze manufacturing data. We asked MaiLab’s young developers to share its concept and potential.
Against a backdrop of issues arising from the retirement of older, skilled staff and the insufficient availability of workers to replace them, many manufacturing sites are upgrading their machines and processes for smart production. One of the basic principles of smart production is to collect as much data as possible from the live production processes and to use the results of analysis to improve the efficiency and productivity of on-going operations.
In order to use the production data, it is necessary to have two sets of skills; the first is to be able to analyze the large volumes of accumulated data, and the second is to apply the results of the analysis back to the actual manufacturing site, which requires overall production site know-how and an understanding of how manufacturing equipment actually works.
However, experience shows that almost no manufacturing sites have this combination of skills in sufficient strength, and furthermore that most enterprises are perplexed about how to use their accumulated data.
To help address this problem, Mitsubishi Electric has developed the data science tool MELSOFT MaiLab (MaiLab) to analyze the data coming from manufacturing sites and processes. It is based on the concept that everyone should be able to easily make use of data and utilizes artificial intelligence (AI) to analyze and suggest ways to address the issues identified.
The MaiLab software was developed by young engineers in their 20s. This was a deliberate decision by Mitsubishi Electric in order to utilize their perspectives on what was required.
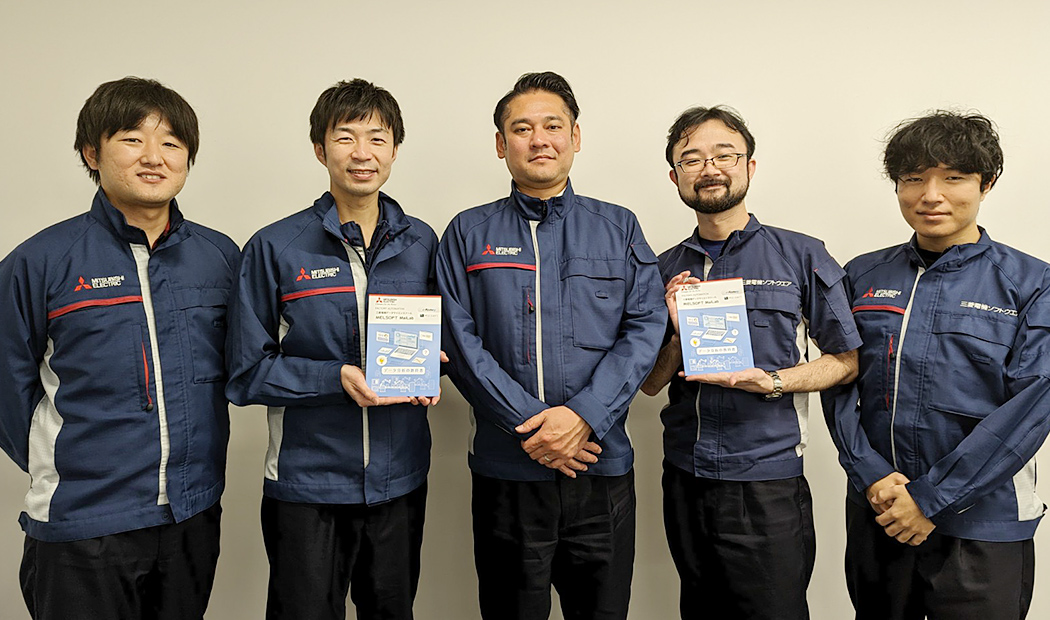
The MaiLab development team, all of whom are in their early 20s. From the left;
Development team leader: Takanori Eguchi, Software System Dept., Nagoya Works. Subleader Shota Nakano, from the same section as Mr. Eguchi.
Sales and marketing: Yoshihiro Ishihara, Sales Dept., Nagoya Works.
From the software development team: Tetsuya Yamada, group leader of FA Engineering Development Dept., Mitsubishi Electric Software Corporation, and Hiroshi Ito also from the same group as Mr. Yamada.
Mitsubishi Electric has a long history of data application technologies and solutions having launched its e-F@ctory concept as far back as 2003. This provided an important mechanism for collecting data from various pieces of equipment on the manufacturing floor, such as programmable controllers, sensors and switches, by using an ICT (Information and communication technology) network. Analysis of this data can then be used to develop plans for cost reductions and increased productivity.
However, in reality the installation of such data capture processes has often led to employees admitting that although they have collected the data, they do not understand how to view or handle it. This does not mean that analyzing the data to produce useful, practical results is impossible. It means they do not know how to do the analysis. Realizing this led Mitsubishi Electric to identify the need for a tool that could enable anyone to easily analyze data.
Mr. Takanori Eguchi, the MaiLab development team leader from Mitsubishi Electric Nagoya Works, Software System Dept., explains “Until now, data analysis has been considered a difficult subject, reserved only for highly qualified professional experts like data scientists and computer engineers. We, somehow, felt that this hurdle could be overcome by using advanced digital technologies such as AI.”
He explains that the project team set the development goal of producing a tool that enables anybody who is involved in FA (factory automation) to do data analysis through the ‘democratization of AI’.
Mr. Eguchi says that while developing a new data science tool, the first thing to focus on is the design. “Earlier generation tools used in FA were designed with an emphasis on the functional aspects. This meant that to use them you had to have a good understanding of how they worked and what else they could do that was not being used. They were not something which could be understood easily by anyone other than a specialist.”
“Significantly, in recent years, an increasing number of young designers have become accustomed to the sort of intuitive interfaces used with smartphone applications, tablets and other consumer technologies. This has had the consequence of widening the useability gap with conventional tools and interfaces.”
This realization led the MaiLab team to prioritize improvements in the design of their user interface, so that it became more like today’s consumer technology.
Mr. Eguchi continues, “In addition to the design, it was decided to develop MaiLab as a web application. That meant the development language would also be changed, and to meet that challenge, we realized we needed a team of young staff members, so we selected people who were all in their 20s.”
The MaiLab development project was started in 2020, that is to say during the Covid pandemic. Team member Mr. Tetsuya Yamada from Mitsubishi Electric Software Corporation (MESW) recalls: “The work was progressing slowly as we fumbled around in the initial phase of development. But eventually we succeeded in creating an environment where everyone could cooperate in the development, whether from home or at work and so the project speeded up considerably.”
“The MaiLab project was finally completed in just two years, and released in July 2022.”
On-site data analysis focused on usability
So how does MaiLab work in the real world? MaiLab is a tool which simplifies data analyses and handles it in four basic steps. These are: creation of a data set, selection of an AI methodology, creation of a task, and task execution and monitoring.
By utilizing AutoML (automated machine learning) functions, the built-in AI Data Scientist automatically selects the pre-processing and analysis methods. It then starts processing the dataset and runs until the analysis is complete. The methods of analysis included deep learning, random forest, multiple regression analysis, MT method, and k-NN (nearest neighbor) method.
Mr. Eguchi highlighted that the team consciously avoided the use of specific terms or jargon associated with data analysis. That way even users unfamiliar with data analysis can complete the setup correctly by simply answering easy questions such as “What is the purpose?” and “Does the data set include any error values?.”
“The point is that the questions are set in such a way that production engineers at the manufacturing site can easily understand them.”
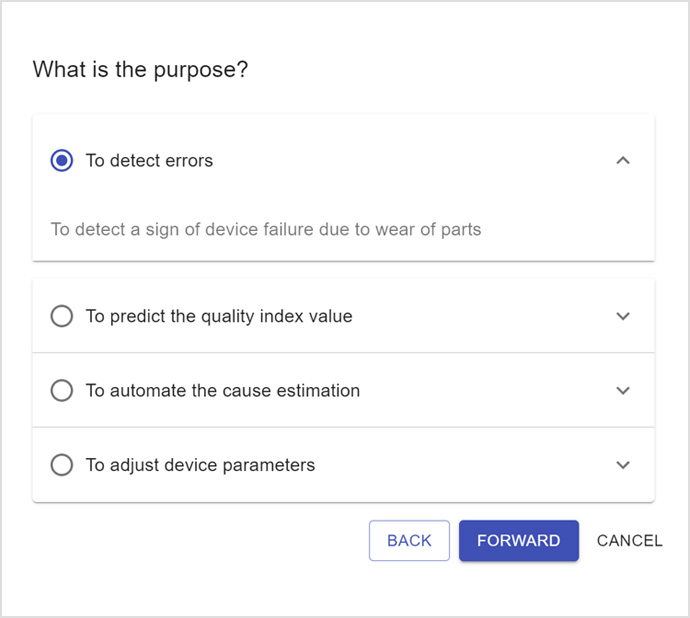
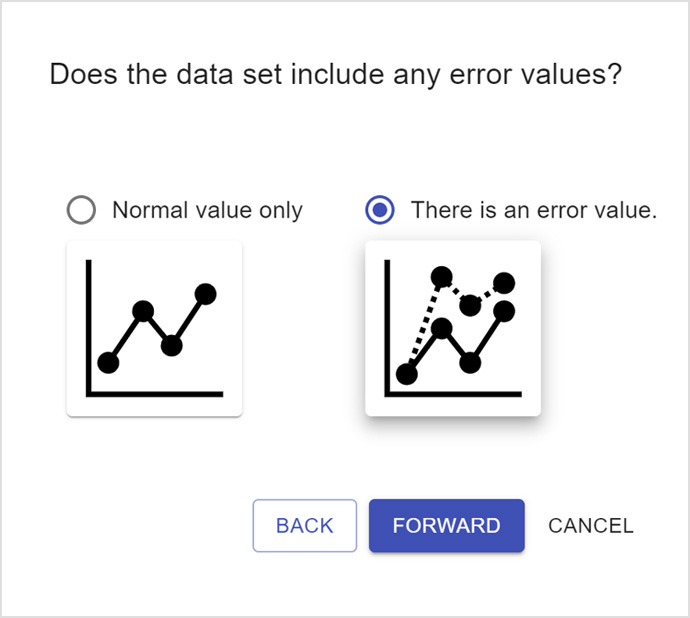
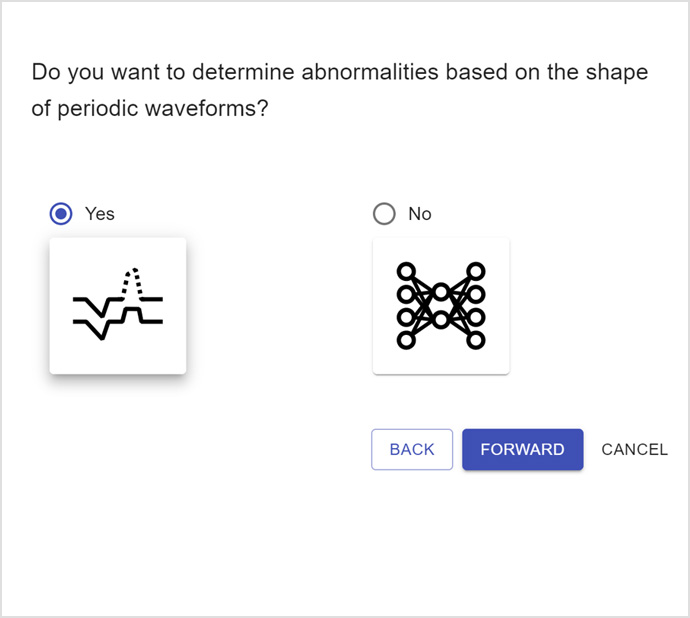
The built-in AI Data Scientist can automatically select the best method for analysis of the data set by asking the user simple, straight-forward questions.
The collection of data from manufacturing equipment can also be easily done. If Mitsubishi Electric PLCs are being used, then it is easy to collect data over Ethernet or with simple steps using external memory. Where the PLC or manufacturing equipment is made by another company, it is possible to use the data collected using a simple procedure based on CSV Files.
One aspect the development team was keen to create was an ‘ultra-cool’ design. This was to include an intuitive interface that beginners would be happy to start using immediately. Mr. Hiroshi Ito, also from Mitsubishi Electric Software Corporation (MESW) explains that the interface emphasizes visibility so that it can be understood at a glance. He explains, “We deliberately avoided packing too many items into the screen-space by prioritizing a layout that promoted clarity. The bottom line is, we created an intuitive and easily understood interface, which is the major component of the ultra-cool design.”
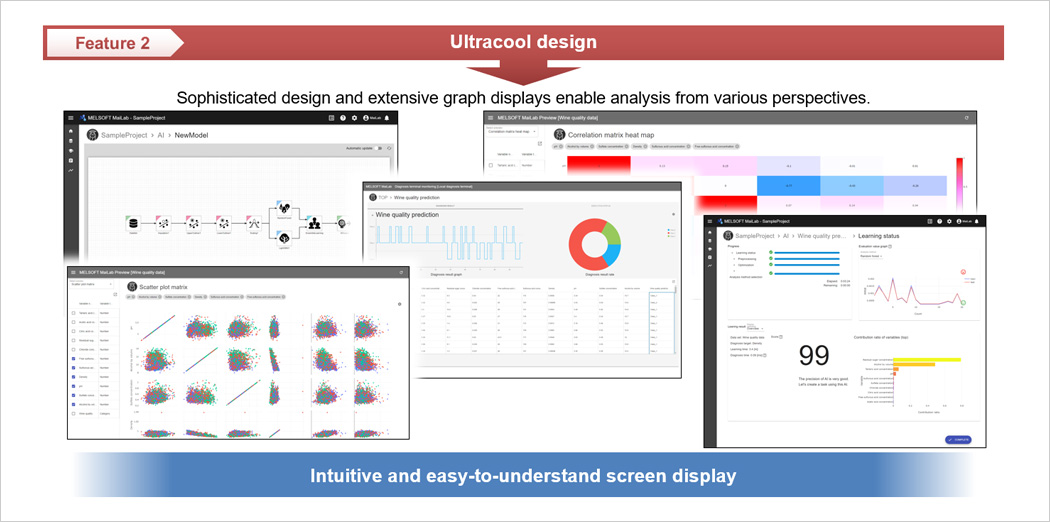
Intuitive interface, clear design.
What does MaiLab deliver?
Developing a new product is only half the challenge. It also must be introduced to users and show them some real benefits. So how did this stage in the MaiLab project go?
Team member Mr. Yoshihiro Ishihara, Mitsubishi Electric Nagoya Works, Sales Dept., runs through the details, “We set up a 90-day trial program for customers to experience MaiLab. There were 750 downloads of MaiLab in the first six months alone, so I feel that we are getting good results.”
“An early finding has been that MaiLab users are using it to identify potential cost reductions at their manufacturing sites.,” he explains.
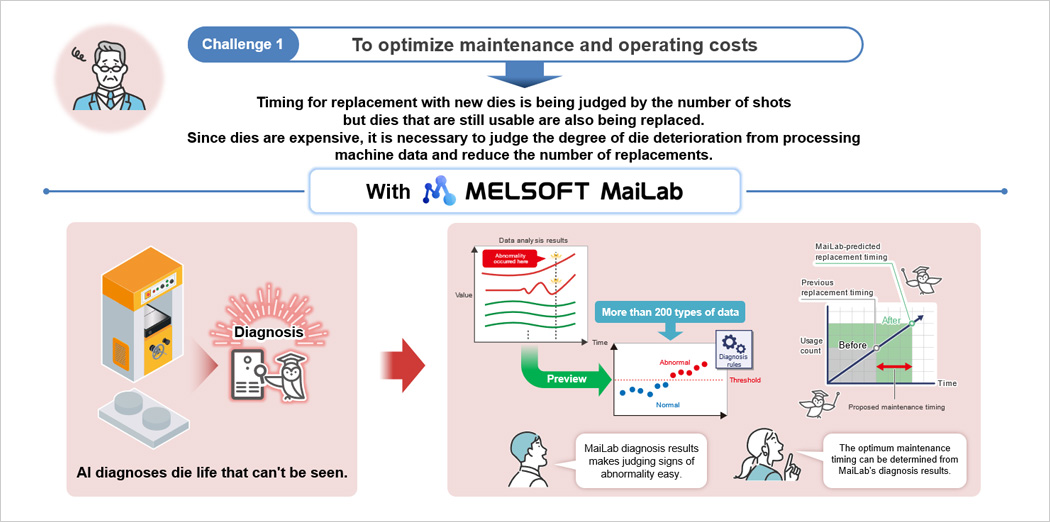
MaiLab proposes optimal tool replacement timings.
One trial participant, a machine tool user, applied MaiLab to a long-standing issue. Since cutting tools and molds for use on machines are expensive, they need to be used for as long as possible, almost until the very end of their life. However, if the tool breaks or the mold fails, defective products may be produced, raw materials could be wasted, and finally the production line will need to stop. This is potentially costly and time consuming, and the knock-on effects could be major.
In the past there was difficulty in determining accurate replacement timings, and it proved prudent to change the tool or mold well before the end of its life using simple evaluations like the number of times the tool or die had been used. This avoided problems and disruption from unplanned downtime but introduced the possibility of significant cost from the loss of the tool or mold’s remaining lifespan.
By using MaiLab, it has become possible to easily build a system to diagnose signs of abnormality with the tool or mold, which are difficult to detect with the human eye. The condition of the tool or mold is quantified using multiple parameters related to the equipment such as motor vibration, current value, pressure, and ambient temperature, and then comparing it with the data from normal operations. By monitoring for signs of abnormality, a timely warning about the need for replacement parts or the need for maintenance can be given before any failure or accident occurs. Therefore, the cost of maintenance and operations can be optimized by proposing an appropriate time for replacement depending on the condition, instead of deciding a threshold value from the beginning. Importantly this arrangement was simply set up by answering the questions in the MaiLab user interface.
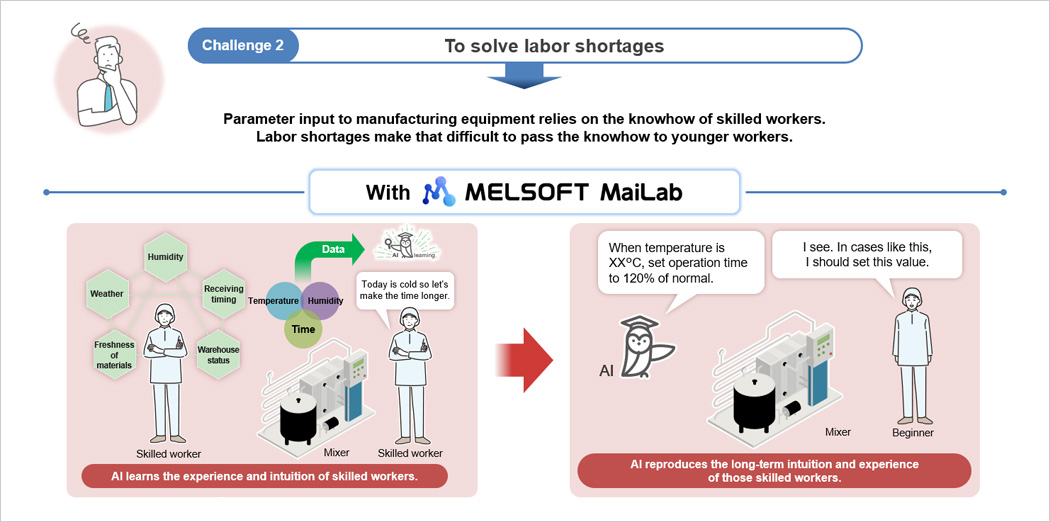
MaiLab learns and reproduces the intuition and experience of skilled engineers.
Optimizing operations with MaiLab
Another typical MaiLab application example, which responds to a current issue in Japan and many other developed industrialized economies, is how to counter the issues of skills loss and shortages of human resources. In the food industry for example, there are many variable parameters, from the ingredients used to the required cooking conditions such as temperature, humidity, cooking times, and portion size, etc. It is extremely difficult to determine optimized set-up values for any given set of these variables, and often skilled food production engineers maintain the quality of the product by making their own intuitive changes to the actual manufacturing process. In the majority of food plants this know-how is rarely documented and takes time for younger engineers to acquire these skills.
MaiLab is able to replicate this longstanding, intuitive experience. Firstly, it collects multiple sets of time-based production data such as fermentation times, temperatures, and the timing of when ingredients are added from various sensors around the machines and their process parameters. As a result, MaiLab’s AI analysis can then be used to guide young engineers when fine tuning the production process in real time to achieve similar results to those of a skilled engineer with many years’ experience.
Mr. Eguchi says, “In the past, at many manufacturing sites years of knowledge were lost every time a long-standing engineer retired or left. By allowing AI to learn this knowledge, it becomes the company’s property, and it can be passed on to other personnel.”
He continued, “It is also useful for producing training materials to explain what points or groups of points experienced engineers evaluate during machine adjustments. It can even be used to overcome issues arising from shortages of human resource or lack of successors.”
Data-centric environments
MaiLab is now available via an annual license system which also means users can benefit from regular feature updates. For example, in order to improve connectivity, Mitsubishi Electric is working on expanding OPC UA (OPC Unified Architecture) connectivity and its support for connecting databases.
Mitsubishi Electric sees MaiLab as a key element towards the digital transformation of industries, and plans to create a data-centric ecosystem by building deep collaboration with the GENESIS64 SCADA (Supervisory Control and Data Acquisition) software, and the 3D simulator “MELSOFT Gemini”.
Sub-leader of the development team, Mr. Shota Nakano explains, “Because it is our first web application, MaiLab’s development follows the trend of digitally connecting data. We faced many challenges in the development process, and there were struggles during the initial stages, but after receiving feedback from the many trial users, we knew that we were heading in the right direction. And I fully expect that in the future we will be applying our MaiLab experiences to other products and solutions.”
In the manufacturing sector where there are specific problems of labor shortages and the transfer of skills and knowledge, data utilization and related systems will have an increasingly important role to play. So, if you are having trouble creating a data-centric system, MaiLab, which makes use of Mitsubishi Electric's extensive knowledge of manufacturing sites, will be of great help.
Reprinted from MONOist article posted on March 14, 2023
https://monoist.itmedia.co.jp/mn/articles/2303/14/news001.html
This article is published with permission from MONOist.
* Affiliation names and positions are as of 2022.